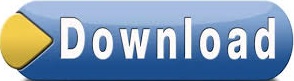

Lin set a regularization parameter for SVM by employing a classification performance. As a result, more minority samples were correctly predicted without compromising the accuracy of the system. Farquad and Bose employed a trained SVM to preprocess imbalanced data. Thus, it is reasonable for decision boundary-based classifiers to employ SVM as a preprocessing method to tackle the problem of imbalanced data. Support vector machine (SVM) is a classification model based on classification decision boundary, and the learned decision hyperplane is only related to support vectors located near the decision hyperplane. Therefore, to obtain informative samples for learning a given classifier, the nature of classification models should be considered before selecting a method for changing the distribution of data. For example, classification models based on classification decision boundary are more dependent on the samples near the decision boundary, whereas classification models based on data distribution are more dependent on the overall and local distribution of the samples. In fact, different classification models have different preferences for samples. This strategy has been featured in recent researches, which placed more emphasis on the availability of each sample, expecting to incorporate as many informative samples for the classifiers as possible in the balanced dataset. The basic objective of this strategy is to change the distribution of datasets and balance the sample size of various classes. This study focuses on the first strategy for handling imbalanced classification problems. Once several balanced datasets were generated, each can be used to learn a classifier that will be later combined into an ensemble classifier. The ensemble classifiers divide the majority samples into several subsets, where each subset has a similar size to the minority class. Costsensitive methods assign higher costs to the minority samples so that the learned classifiers can identify more minority samples. The second strategy encompasses common methods, such as cost-sensitive techniques and ensemble classifiers. Hybrid sampling transforms an imbalanced dataset into a balanced dataset by adding minority samples via oversampling and reducing the majority samples via undersampling. Oversampling selects some samples from the minority class or generates new samples based on the existing minority samples, and then, adds the selected or generated samples into the minority class, thereby obtaining a balanced dataset. Undersampling rebalances an imbalanced dataset by removing part of the samples from the majority class. The first strategy can be further classified into undersampling, oversampling, and hybrid sampling methods, which are used to change the class distribution. The first strategy is to change the distribution of various classes in a dataset, and the second is to design or modify learning algorithms to reduce the negative effect of class imbalance. Researchers in the field of imbalanced classification problems have long been focusing on improving the recognition rate of minority samples.Ĭurrently, two main strategies are used to address classification problems with imbalanced data.

Detecting rare events is, in essence, the process of identifying samples in a minority class from an imbalanced dataset. Rare events (such as cancer in cancer detection, financial fraud in financial fraud detection, and intrusion events in intrusion detection ) are usually difficult to detect owing to their relative scarcity however, detecting rare events is more critical than detecting ordinary events in many practical problems.

Keywords: Imbalanced Data, Safe Sample Screening, Re-SSS-IS, Re-SSS-WSMOTE The experimental results show that Re-SSS can effectively improve the classification performance of imbalanced classification problems. Both Re-SSS-IS and Re-SSS-WSMOTE are based on safe sampling screening. The Re-SSS-IS selects informative samples from the majority class, and determines a suitable regularization parameter for SVM, while the Re-SSS-WSMOTE generates informative minority samples. This study developed a resampling algorithm for Rebalancing imbalanced data using Safe Sample Screening (Re-SSS), which is composed of selecting Informative Samples (Re-SSS-IS) and rebalancing via a Weighted SMOTE (Re-SSS-WSMOTE).
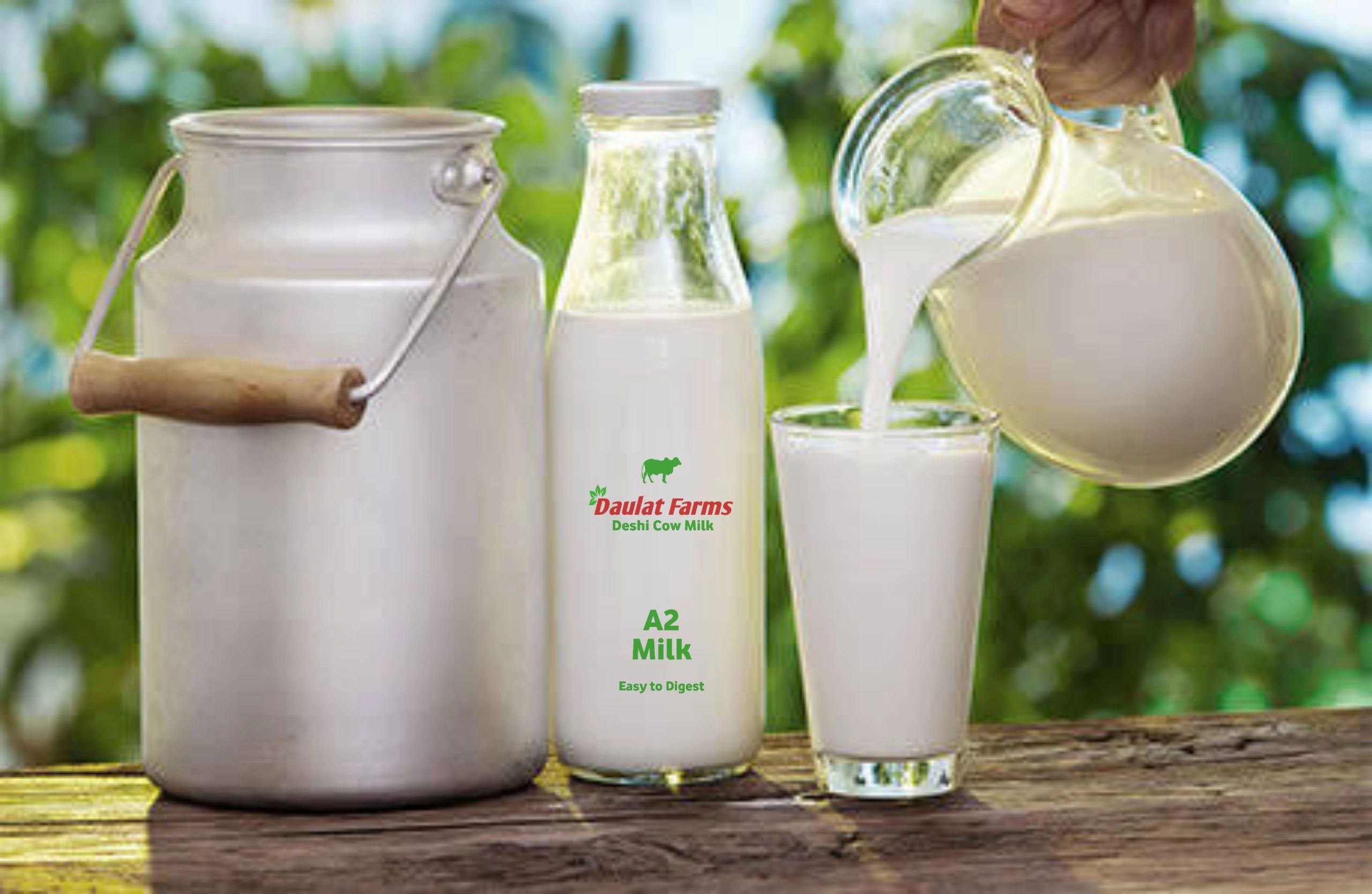
Safe sample screening can identify a part of non-informative samples and retain informative samples. To rebalance an imbalanced dataset, it is reasonable to reduce non-informative samples and add informative samples for learning classifiers. Abstract: Different samples can have different effects on learning support vector machine (SVM) classifiers.
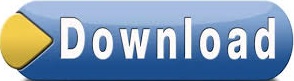